hdfs系列 – Text格式与mapreduce
入门hadoop,我认为很重要也很困难的一点就是理解各种纷杂的文件格式与压缩算法的关系,理解mapreduce如何与不同的文件格式进行合作,只有建立好这些认知才能进一步用好hive等上层组件。
hdfs系列分为4篇,将演示text、sequence、Avro、Orc(列存储)共4种文件格式的hdfs读写与mapreduce开发,经过学习可以加深对文件格式、压缩算法、mapreduce的理解,代码链接如下:
- HDFS示例代码:https://github.com/owenliang/hdfs-demo。
- Mapreduce示例代码:https://github.com/owenliang/mr-demo。
本文将讲解带压缩的text文本格式。
mvn依赖
引入hadoop依赖:
1 2 3 4 5 |
<dependency> <groupId>org.apache.hadoop</groupId> <artifactId>hadoop-client</artifactId> <version>3.3.0</version> </dependency> |
增加maven-shade-plugin,指定main类并且让依赖一起打包到jar中:
1 2 3 4 5 6 7 8 9 10 11 12 13 14 15 16 17 18 19 20 21 22 23 24 25 |
<build> <plugins> <plugin> <groupId>org.apache.maven.plugins</groupId> <artifactId>maven-shade-plugin</artifactId> <version>3.2.4</version> <executions> <execution> <phase>package</phase> <goals> <goal>shade</goal> </goals> <configuration> <transformers> <transformer implementation="org.apache.maven.plugins.shade.resource.ManifestResourceTransformer"> <Main-Class>cc.yuerblog.Main</Main-Class> </transformer> </transformers> </configuration> </execution> </executions> </plugin> </plugins> </build> |
HDFS程序
访问HDFS需要new一个Configuration配置对象,通常我们不需要主动set任何配置,稍后运行程序时它会自动加载hadoop环境变量。
1 2 3 4 5 |
Configuration conf = new Configuration(); try { // 无格式文件 RawFileTest raw = new RawFileTest(); raw.run(conf); |
我们直接打开HDFS文件,写入\n换行的文本,并采用gzip编码压缩数据,说白了就是在HDFS上写入一个gzip压缩后的文本文件而已。
1 2 3 4 5 6 7 8 9 10 11 12 13 14 15 16 17 18 19 20 21 22 23 24 25 26 27 28 29 30 31 32 33 34 35 36 37 38 39 40 41 42 43 44 45 46 47 48 49 50 |
package cc.yuerblog.fs; import org.apache.hadoop.conf.Configuration; import org.apache.hadoop.fs.FSDataInputStream; import org.apache.hadoop.fs.FSDataOutputStream; import org.apache.hadoop.fs.FileSystem; import org.apache.hadoop.fs.Path; import org.apache.hadoop.io.compress.CompressionCodec; import org.apache.hadoop.io.compress.CompressionCodecFactory; import org.apache.hadoop.io.compress.CompressionInputStream; import org.apache.hadoop.io.compress.CompressionOutputStream; import java.io.BufferedReader; import java.io.IOException; import java.io.InputStreamReader; public class RawFileTest { public void run(Configuration conf) throws IOException { // HDFS FileSystem dfs = FileSystem.get(conf); // 文件路径 Path path = new Path("/raw.gz"); // GZIP编码器 CompressionCodec codec = new CompressionCodecFactory(conf).getCodecByName("gzip"); // 写打开 FSDataOutputStream out = dfs.create(path); // 压缩编码流 CompressionOutputStream codecOut = codec.createOutputStream(out); // 写入数据 for (int i = 0; i < 1000; i++) { codecOut.write("hello world\n".getBytes()); } codecOut.close(); // 读打开 FSDataInputStream in = dfs.open(path); // 压缩解码流 CompressionInputStream codecIn = codec.createInputStream(in); // 按行读 BufferedReader bufIn = new BufferedReader(new InputStreamReader(codecIn)); while (bufIn.ready()) { String line = bufIn.readLine(); System.out.printf("[Raw] %s\n", line); } bufIn.close(); } } |
FileSystem是HDFS的抽象,Path是路径的抽象,CompressionCodec是压缩编码器的抽象。
dfs.create创建文件并清空它,返回一个FSDataOutputStream输出字节流,通过codec.createOutputStream包装原始输出流得到一个压缩字节流,然后向codecOut逐行写出数据即可。
dfs.open读打开一个文件,发挥一个FSDataInputStream输入字节流,通过codec.createInputStream包装原始输入流得到一个解压字节流。
为了方便逐行读取,利用InputStreamReader包装字节流输入,得到字符流输入,并进一步包装给BufferedReader,从而可以调用readLine()方法解析成行文本。
注意,这里写入的文件名叫做raw.gz,采用压缩类型对应的标准文件后缀是很重要的,因为后续Mapreduce解析该文件时会通过文件后缀进行自动解压。
运行hdfs程序
将mvn package得到的jar包上传到安装了hadoop环境的服务器,利用hadoop脚本的jar命令执行程序,这样Configuration才可以加载到对应hadoop集群的配置:
1 2 3 |
root@ubuntu:~/bigdata/demo# hadoop jar Usage: hadoop jar <jar> [mainClass] args... root@ubuntu:~/bigdata/demo# hadoop jar hdfs-demo-1.0-SNAPSHOT.jar |
也可以使用hdfs命令查看raw.gz文件的内容:
1 2 3 |
root@ubuntu:~/bigdata/demo# hdfs dfs -cat /raw.gz �Ʊ ��)Nዃw ��J����5�������������������������;�'��.r |
发现cat命令打印了乱码,因为它是压缩后的文件,可以通过-text命令让hdfs命令自动识别压缩类型进行解压:
1 2 3 4 5 6 7 8 |
root@ubuntu:~/bigdata/demo# hdfs dfs -text /raw.gz hello world hello world hello world hello world hello world hello world hello world |
Mapreduce原理
HDFS文件默认64MB一个block分块存储,这样可以启动多个mapper并发读取不同的block进行运算。
如果数据量不足一个block,其实对于这种\n分隔符的文件,通过TextInputFormat文件格式也将通过\n找到一些分隔点,从而让N个mapper并发处理1个block的不同部分。
然而上述raw.gz是gzip压缩过的文本文件,TextInputFormat无法从压缩后的数据中找到\n,因此也就无法划分文件给多个mapper并发处理,因此这种普通文本文件经过压缩后是无法发挥mapper并发能力的,处理速度会很慢。
Mapreduce框架有几个关键概念来抽象”文件格式”、”文件分片”、”记录读取”:
InputFile一定是按某种文件格式写入的,通过InputFormat可以对其进行解析,通过寻找切分边界可以将整个文件切分成多个分片叫做InputSplit,每个InputSplit对应文件的一个片段,通过对应的RecordReader对象可以逐个record的进行获取,mapper最终访问的就是recordReader来获取<K,V>输入的。(文件行原本没有KV概念,但是MR要求InputFormat最终喂进去的是KV,所以InputFormat的RecordReader都要遵循MR的要求去搞成KV输入)
因此,无论任何文件格式的实现,其RecordReader都要按<K,V>格式将解析到的一行数据交给Mapper,具体K和V的含义则是对应文件格式自行约定的,我们需要看具体文件格式的文档说明,大家可以看这篇文章了解一下各种文件格式:https://www.doudianyun.com/2018/11/mapreduce-inputformat/
mapreduce程序
我们实现MR统计一下raw.gz中一共有多少行hello world。
先创建configuration来加载hadoop集群的配置信息:
1 2 3 4 5 6 7 |
public static void main(String[] args) { Configuration conf = new Configuration(); try { // 文本文件(带压缩)MR RawMR rawMR = new RawMR(); rawMR.run(conf); |
在内嵌类实现Mapper和Reducer:
1 2 3 4 5 6 7 8 9 10 11 12 13 14 15 16 17 18 19 20 21 22 23 24 25 26 27 28 29 30 31 32 |
package cc.yuerblog.mr; import org.apache.hadoop.conf.Configuration; import org.apache.hadoop.fs.FileSystem; import org.apache.hadoop.fs.Path; import org.apache.hadoop.io.LongWritable; import org.apache.hadoop.io.Text; import org.apache.hadoop.io.compress.GzipCodec; import org.apache.hadoop.mapreduce.Job; import org.apache.hadoop.mapreduce.lib.input.TextInputFormat; import org.apache.hadoop.mapreduce.lib.output.TextOutputFormat; import java.io.IOException; public class RawMR { private static class Mapper extends org.apache.hadoop.mapreduce.Mapper<LongWritable, Text, Text, LongWritable> { @Override protected void map(LongWritable key, Text value, Context context) throws IOException, InterruptedException { context.write(value, new LongWritable(1)); // (内容,1次) } } private static class Reducer extends org.apache.hadoop.mapreduce.Reducer<Text, LongWritable, Text, LongWritable> { @Override protected void reduce(Text key, Iterable<LongWritable> values, Context context) throws IOException, InterruptedException { long count = 0; for (LongWritable v : values) { count += v.get(); } context.write(key, new LongWritable(count)); } } |
Mapper的输入是<文件偏移量,行内容>,输出是<行内容,1>;Reducer的输入是<行内容,1>,输出是<行内容,总次数>。
Mapper和Reducer的K必须实现WritableComparable接口,因为K首先要在mapreduce的shuffle过程中序列化到文件与网络传输,其次K必须可以比较,因为shuffle过程中相同的K会排序到一起。V则只需要实现Writable序列化能力即可,这样shuffle阶段value可以被写入文件与网络传输。
所以在Mapper和Reducer的template参数中,我们使用的都是Writable的Text和Long,而不是原生JAVA类型。
1 2 3 4 5 |
public interface Writable { void write(DataOutput var1) throws IOException; void readFields(DataInput var1) throws IOException; } |
K和V只要可序列化即可,因此可以是Protoclbuf或者Avro等序列化协议,其实都是无所谓的,不过一般我们就用hadoop内置的一些Writable类型实现。
接下来需要配置一下MR job,这块的工作比较多而且都是必须的:
1 2 3 4 5 6 7 8 9 10 11 12 13 14 15 16 17 18 19 20 21 22 23 24 25 26 27 28 29 30 |
public void run(Configuration conf) throws Exception { Path input = new Path("/raw.gz"); Path output = new Path("/raw-mr"); // 删除之前的结果 FileSystem.get(conf).delete(output, true); // 创建Job Job job = Job.getInstance(conf, "RawMR"); job.setJarByClass(RawMR.class); // mapper/reducer实现在该类内部,需要设置这个 /// 输入 TextInputFormat.addInputPath(job, input); // 文件路径 job.setInputFormatClass(TextInputFormat.class); // 文件格式 job.setMapperClass(Mapper.class); // mapper实现 job.setMapOutputKeyClass(Text.class); // 中间key job.setMapOutputValueClass(LongWritable.class); // 中间value // 输出 TextOutputFormat.setOutputPath(job, output); // 文件路径 TextOutputFormat.setCompressOutput(job, true); // 开启压缩 TextOutputFormat.setOutputCompressorClass(job, GzipCodec.class); // gzip压缩 job.setOutputFormatClass(TextOutputFormat.class); // 文件格式 job.setReducerClass(Reducer.class); // reducer实现 job.setOutputKeyClass(Text.class); // 结果key job.setOutputValueClass(LongWritable.class); // 结果value // 等待任务执行,打印详情 job.waitForCompletion(true); } |
首先需要删除上次MR的输出目录,并且设定MR程序所在的class。
我们使用TextInputFormat,它对.gz压缩文件只会启动1个mapper顺序解码gzip文件,我们配置了若干输入选项:
- addInputPath:输入路径,可以是目录
- setInputFormatClass:该文件类型的RecordReader会给Mapper输入<文件偏移量,文本行内容>
- setMapperClass:mapper实现
- setMapOutputKeyClass:mapper输出的K类型
- setMapOutputValueClass:mapper输出的V类型
mapper输出的K,V经过shuffle后,相同K会被分配给同1个reducer,这个过程K和V采用MR框架内置的格式进行中间文件组织与存储,我们只需要关心K和V自身的序列化即可。
输出仍旧采用Text格式,配置了若干输出选项:
- setOutputPath:reducer输出目录
- setCompressOutput:reducer输出内容开启压缩
- setOutputCompressorClass:reducer输出采用gzip压缩
- setReducerClass:reducer实现
- setOutputKeyClass:reducer输出K的类型
- setOutputValueClass:reducer输出V的类型
最后等待任务执行即可。
运行mapreduce
mvn package把jar包上传hadoop服务器,然后执行:
1 |
hadoop jar mr-demo-1.0-SNAPSHOT.jar |
查看输出目录:
1 2 3 4 |
root@ubuntu:~/bigdata/demo# hdfs dfs -ls /raw-mr Found 2 items -rw-r--r-- 1 root supergroup 0 2020-10-07 02:40 /raw-mr/_SUCCESS -rw-r--r-- 1 root supergroup 37 2020-10-07 02:40 /raw-mr/part-r-00000.gz |
默认我们没有指定reducer数量(可以通过 job.setNumReduceTasks方法指定),所以只启动了1个reducer输出了1个分区的文本文件,采用.gz压缩,查看其内容:
1 2 |
root@ubuntu:~/bigdata/demo# hdfs dfs -text /raw-mr/part-r-00000.gz hello world 1000 |
发现TextOutputFormat实际把reducer的K,V输出为了\t分隔的文本行。
实际情况中,日志采集写入hdfs后可能按小时压缩成.gz,多个采集器写入不同的.gz文件,这样mapreduce可以为每个.gz创建1个mapper来提升并发度。
另外,Mapreduce清洗后输出的格式一般不会继续采用文本格式+gz压缩,因为后续往往会交给hive数仓进一步SQL分析,因此往往这里的输出将采用更高级的文件格式来支持压缩情况下的文件分片能力,以便hive能够获得更好的mapper计算并发度,在后面的文件格式我们会具体分析这些高级文件格式。
如果文章帮助您解决了工作难题,您可以帮我点击屏幕上的任意广告,或者赞助少量费用来支持我的持续创作,谢谢~
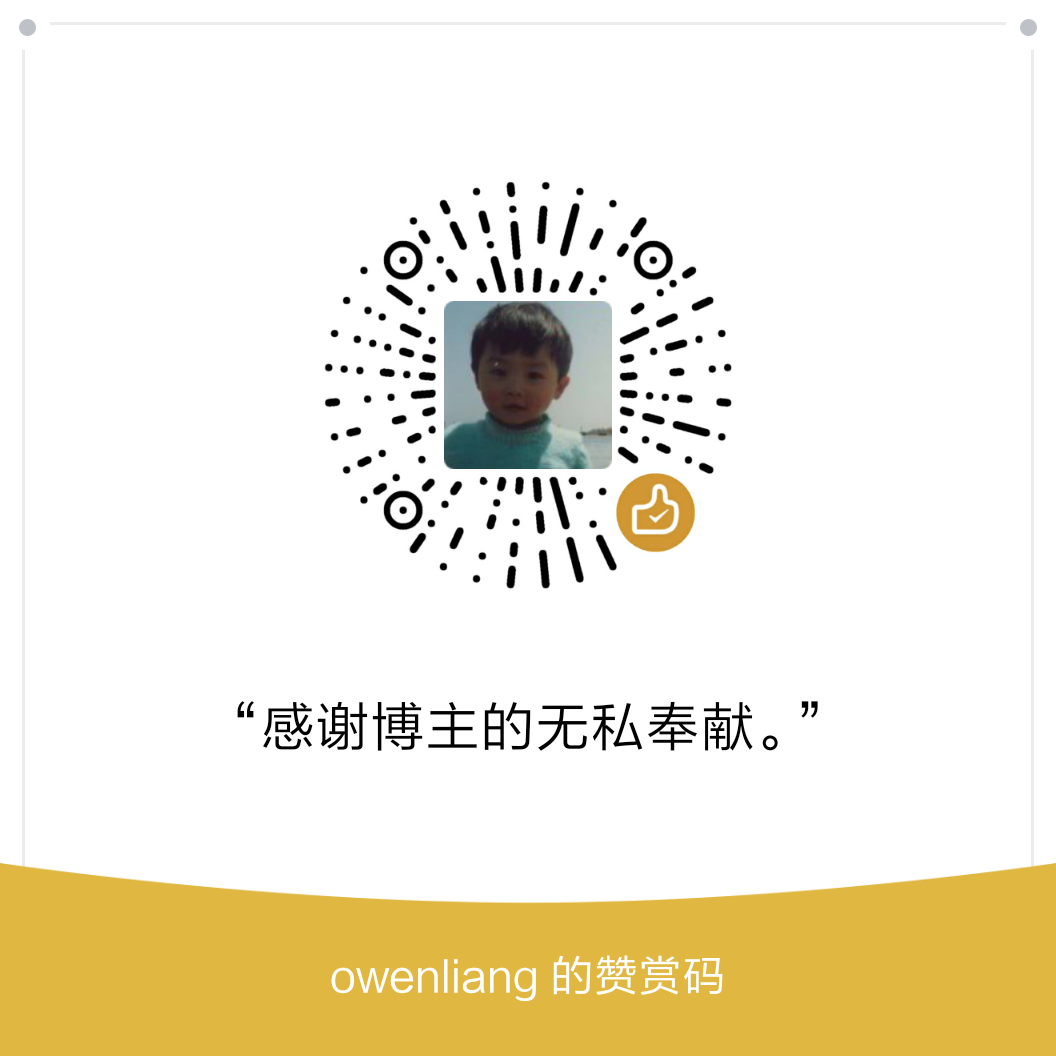
One thought on “hdfs系列 – Text格式与mapreduce”
Comments are closed.